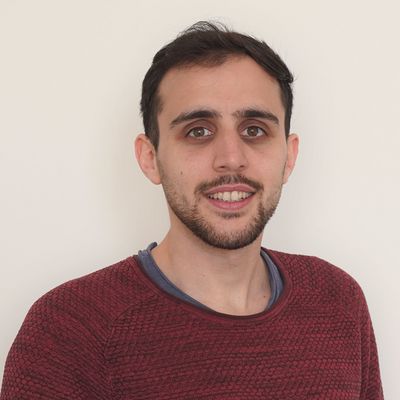
Nabil Jabareen
Charité
Contacts
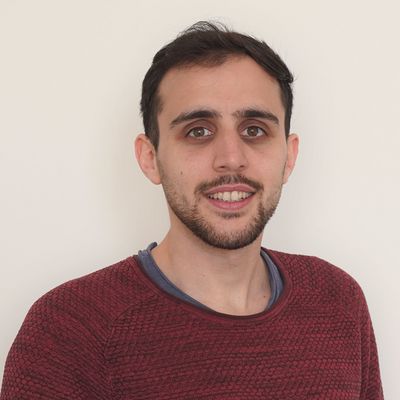
Supervisors:
Sören Lukassen (Charité Universitätsmedizin)
Roland Eils (Charité Universitätsmedizin)
Glioblastoma is the deadliest type of brain cancer. The goal of this project is to help clinicians treat Glioblastoma patients in a way that not only maximizes their survival time, but also improves their quality of life.
To treat Glioblastoma patients, a tri-modal therapy including surgery, Radiation Therapy (RT) and chemotherapy is prescribed. The effectiveness of the surgery and RT is heavily dependent on the analysis and quality of the obtained medical images. By improving Computer-Assisted Interventions (CAI) for image guided RT in brain tumor patients, we aim to improve the effectiveness of RT in Glioblastoma.
To enable CAI using Deep Learning (DL) methods, a large amount of labelled data is necessary. These labels can only be generated by medical experts and are not only time consuming and expensive to generate, but they can also be subjective or even erroneous. Using Self-Supervised Learning (SSL) methods, we use the intrinsic information of medical images as artificial labels to train large DL methods. This enables us to train on a large amount of data and mitigate and explore potential biases of the model. Based on the pre-trained SSL models, we will automate the time consuming and error prone tasks of Target Volume (TV) and the Organ At Risk (OAR) segmentation. Additionally, we will build an interpretable DL model to predict the settings of the RT and the absorbed radiation dose. This model will enable clinicians to experiment with a wide range of RT settings to better personalize the treatment for a given patient.
Peer-reviewed Publications (journal or conference)
- F.W. Ten, D. Yuan, N. Jabareen, Y.J. Phua, R. Eils, S. Lukassen, and C. Conrad (2023). resVAE ensemble: Unsupervised identification of gene sets in multi-modal single-cell sequencing data using deep ensembles. Frontiers in Cell and Developmental Biology, 11, 104.
https://doi.org/10.3389/fcell.2023.1091047 - N. Jabareen and S. Lukassen (2022). Segmenting Brain Tumors in Multi-modal MRI Scans Using a 3D SegNet Architecture. In: Crimi, A., Bakas, S. (eds) Brainlesion: Glioma, Multiple Sclerosis, Stroke and Traumatic Brain Injuries. BrainLes 2021. Lecture Notes in Computer Science, vol 12962. Springer, Cham. https://doi.org/10.1007/978-3-031-08999-2_32
Other (presentations at conferences or preprints)
-
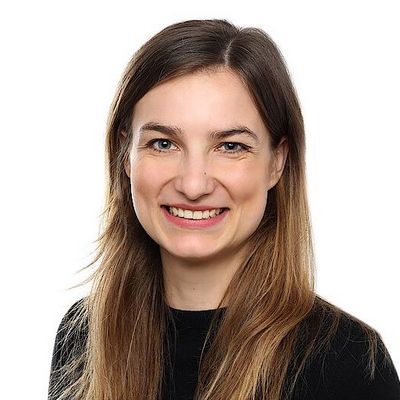
Alexandra Kapp
TU Berlin - HTW
Contact
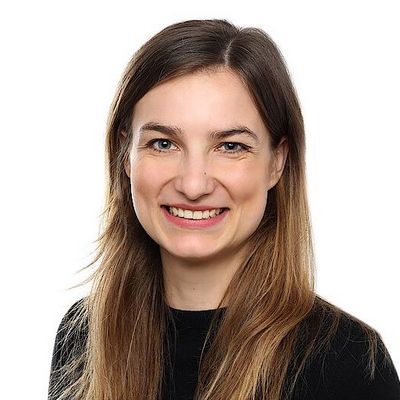
Supervisors:
Human mobility data is a crucial resource for urban mobility applications, such as city planning, traffic modeling, routing applications, or mobility services. Mobility data can bring valuable benefits, but it does not come without personal reference. The implementation of measures such as anonymization is thus needed to protect individuals' privacy. Naturally, a trade-off between privacy and utility arises as such techniques decrease the data’s utility which potentially limits its use.
This work aims to identify, explore implement and evaluate privacy-preserving techniques for mobility data and their impact on the usability in real-world use cases and datasets. Practitioners will likely only adopt such methods if these do not highly impair practical usage. Also, methods need to be made understandable and they need to be easy to implement by the users in practice. Even though large tech companies, such as Apple, Google, and Microsoft already make use of privacy methods with differential privacy guarantees, there is still a gap between state-of-the-art privacy methods and common practices within the majority of companies.
As the impact on applications’ utility stays unclear, practitioners hesitate to implement such methods. This calls for a set of comprehensible utility metrics that quantify the impact on the utility and make different methods easily comparable. Also, academic research often lacks usable implementations for its theoretical solutions that allow easy reuse of the proposed methods. Lacking resources are therefore another hurdle, as the implementation of complex privacy-preserving methods needs time and expertise.
With this work, I want to contribute to the practical applicability of suitable privacy methods for human mobility data according to state-of-the-art privacy research.
Full-length publications
- A. Kapp (2022). Collection, usage and privacy of mobility data in the enterprise and public administrations. Proceedings on Privacy Enhancing Technologies. DOI 10.2478/popets-2022-0117
- A. Kapp, S. Nuñez von Voigt, H. Mihaljević, and F. Tschorsch (2022). Towards mobility reports with user-level privacy. Journal of Location Based Services, 21 Nov 2022. DOI 10.1080/17489725.2022.2148008
- A. Kapp, J. Hansemeyer, and H. Mihaljević (2023). Generative Models for Synthetic Urban Mobility Data: A Systematic Literature Review. ACM Computing Surveys. https://doi.org/10.1145/3610224
- A. Kapp, and H. Mihaljevic (2023). Reconsidering utility: unveiling the limitations of synthetic mobility data generation algorithms in real-life scenarios. In Proceedings of the 31st ACM International Conference on Advances in Geographic Information Systems (SIGSPATIAL '23), 93, 1–12. https://doi.org/10.1145/3589132.3625661
Conference presentations
-
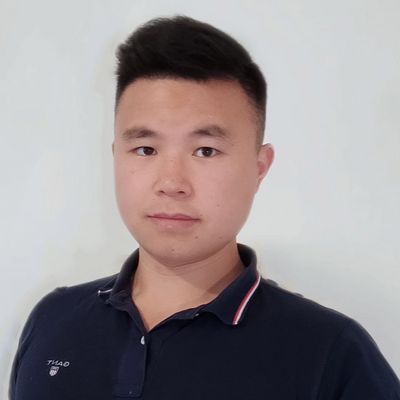
Rui Li
MDC - HZDR - TU Dresden
Contact
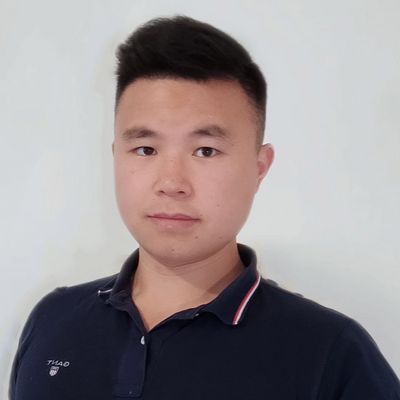
Supervisors:
Artur Yakimovich (HZDR, Dresden)
Ivo F. Sbalzarini (TU Dresden)
3D structure information of biological entities has a strong impact on drug screening and clinical experiments. Microscopy serves as a reliable tool for imaging the 3D structures - both electron microscopy (EM) and light microscopy (LM). On a nanoscale in EM, the Cryo-ET is advancing as a method to determine the biological structure within the entities’ native environment. However, higher time consumption and constraints on electron dose limit the potential of Cryo-ET. On a macro scale in LM, confocal fluorescence microscopy (CFM) obtains axial optical sections by filtering out out-focus light using a pinhole or a slit in the optical path of the microscope. This allows stacking the thin slices into a 3D volume. Yet, CFM comes with drawbacks such as high equipment costs and higher skill requirements in microscopy. In contrast, widefield microscopy is simple and ubiquitous in biomedical laboratories.
Machine learning (ML) serves as a promising end-to-end solution. For 3D model reconstruction in CV, the ML solutions show advantages by restoring 3D information based on limited 2D input images (e.g., single-images and multi-images). In the biology domain, scholars proposed the potential to enhance 3D microscopy performance with ML technology at an early stage. However, since then only few contributions have been made to 3D biological model reconstruction with newly composed ML theories (e.g., GAN, VAE, etc.).
In this work, we will focus on the 3D reconstructions from the focal series of LM and EM using deep neural networks (DNNs). Specifically in Cryo-EM, through electron-optical defocusing we could obtain 3D information of given molecules on the 2D focal planes. We hypothesize it is possible to restore 3D information of pleomorphic objects from 2D images. For LM, instead of the expensive and skills-taxing CFM, we will adopt the images from cheap widefield microscopes. By filtering out the out-focus pixels of images in focal planes through DNNs, We will explore the possibilities to recover 3D information from out-of-focus planes of non-confocal microscopic 3D stacks.
Full-length publications
- R. Li, V. Sharma, S. Thankgamani, and A. Yakimovich (2022). Open-Source Biomedical Image Analysis Models: A Meta-Analysis and Continuous Survey. Frontiers in Bioinformatics. https://doi.org/10.3389/fbinf.2022.912809
- R. Li, M. Kudryashev, and A. Yakimovich (2023). A weak-labelling and deep learning approach for in-focus object segmentation in 3D widefield microscopy. Sci Rep 13, 12275. https://doi.org/10.1038/s41598-023-38490-2
- R. Li, G. della Maggiora, V. Andriasyan, A. Petkidis, A. Yushkevich, M. Kudryashev, and A. Yakimovich (2023). Microscopy image reconstruction with physics-informed denoising diffusion probabilistic model. arXiv. arXiv:2306.02929. [Preprint]
Conference presentations
- L. Rui, M. Kudryashev, and A. Yakimovich. Translate widefield microscopy images into the 3D models in confocal microscope style using deep neural networks. 6th International Symposium on Image-based Systems Biology (ibSB), Online & Jena, Germany, 8-9 September 2022.
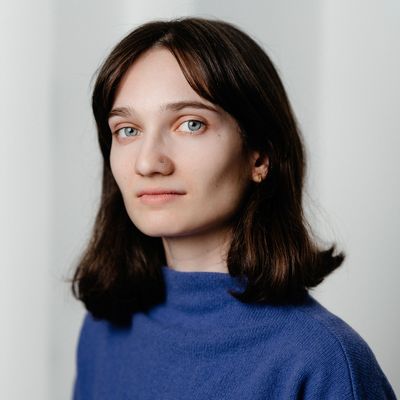
Ana Lomashvili
DLR - TU Berlin
Contact
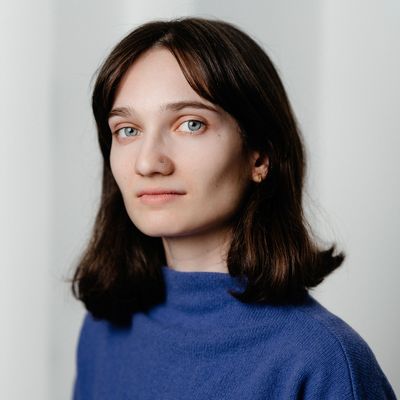
Supervisors:
Understanding the formation and evolution of Mars’ surface could answer such fundamental questions as the habitability of the planet. This motivated multiple ongoing robotics missions including NASA’s rovers "Curiosity" and "Perseverance". These machines are equipped with various measuring tools, providing crucial information to geologists. One of the prominent to this thesis is the ChemCam instrument suite attached to the Curiosity rover to analyze chemical composition of rocks and soils in Gale crater.The instrument consists of two components: the first planetary science Laser-Induced Breakdown Spectrometer (LIBS) and a Remote Micro-Imager (RMI), capturing detailed images of the area illuminated by the laser beam. The instrument pictured already more than 4000 targets and collected LIBS spectra from multiple points of each target (5-25 points per target). The database, consisting of thousands of unlabeled targets, needs to be classified in specific groups with the potential of acquiring information about the geology and environmental conditions on Mars. In this work, the existing rock classification methods will be improved by combining two types of data: LIBS spectra and RMI images.
Full-length publication
-
Conference presentations
- A. Lomashvili, K. Rammelkamp, O. Gasnault, P. Bhattacharjee, E. Clave, C. H. Egerland, S. Schroder, B. Demir, N. L. Lanza. Optimized Martian Dust Displacement Detection Using Explainable Machine Learning. (Poster presentation), IEEE/CVF Conference on Computer Vision and Pattern Recognition Workshops (CVPRW). Seattle WA, USA, 17-21 June, 2024.
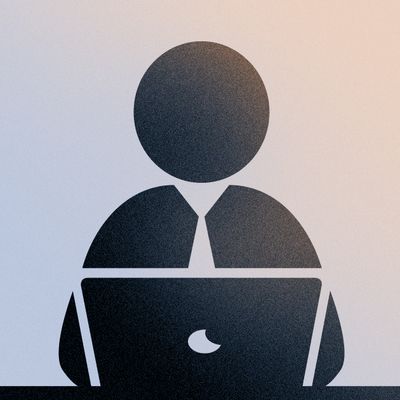
Mark Melzer
Charité
Contact
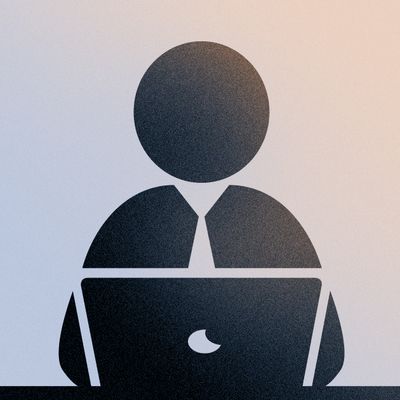
Supervisors:
Lisa Buchauer, Charité Universitätsmedizin
Since the 1970s, flow cytometry has been used in immunology to understand the reaction of the host immune system to diseases. By letting fluorescently marked antibodies bind to their cognate surface molecules and subsequently measuring the antibodies’ abundance via a laser, type and activation state of a cell can be determined. Comparing cell type distributions and activation patterns between different conditions allows to infer knowledge about the immune response to certain stimuli, like viral infections or vaccines. With this setup, flow cytometry has enriched our understanding of the immune system and shaped the development of therapeutic methods.
Over the last decade, the development of spectral flow cytometry has revolutionized the field. The limit for the number of observable markers has increased from around 15 up to 50. In consequence, new challenges arise in the analysis of the data. The classical approach, which relies on human inspection of combinations of two markers at a time and drawing straight lines to separate marker-positive and marker-negative cell populations from each other, quickly becomes too time-consuming and complex for higher dimensional flow cytometry data and, in addition, results are highly dependent on personal choices.
Here, we want to develop a workflow for the construction of flow cytometry atlases comprised of several content-related datasets from different technical sources. To this end, we will first conduct a thorough benchmarking of existing methods; next, by either using the top-performing method or developing our own, construct a proof-of-concept atlas in the area of immune responses to different respiratory diseases; and last, further develop our work into an accessible software solution for research and diagnostic
settings.
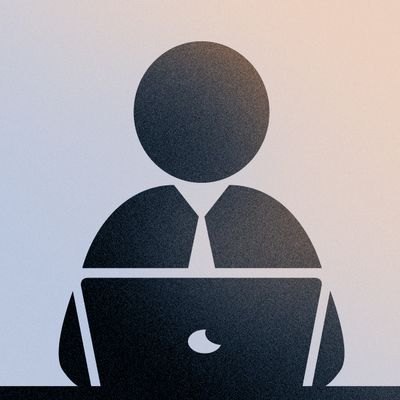
Tancredi Massimo Pentimalli
MDC - Charité
Contact
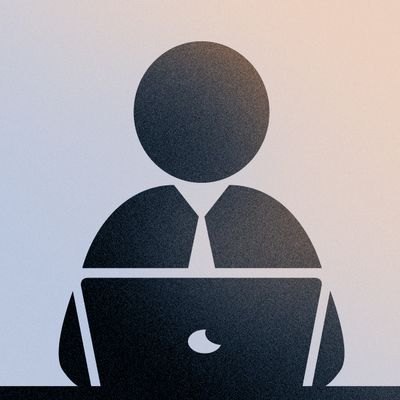
Supervisors:
Angelika Eggert (Charité Universitatsmedizin)
Frederick Klauschen (Charité Universitatsmedizin)
Solid tumors are complex ecosystems, where genetically aberrant malignant cells multiply uncontrolled, stimulate the growth of new blood vessels and remodel the local microenvironment to favour tumor growth, evasion from the immune system and resistance to therapies. Therapeutic targeting of immune inhibitory interactions with the so-called immune checkpoint inhibitors revolutionized the treatment of advanced solid tumors including non-small cell lung cancer and resulted in the award of the 2018 Nobel Prize in Physiology or Medicine to Tasuku Honjo and James Allison. Nevertheless, today only a small fraction of lung cancer patient respond to checkpoint inhibitors. Recent advances in single-cell resolved spatial transcriptomics allow investigating the gene expression and tissue location of thousand of cells in a single experiment, thus providing the unprecedented opportunity to study cell-cell communication between neighboring cells.
In this study, single-cell resolved spatial transcriptomic approaches will be leveraged to dissect the complex interplay between malignant, stromal and immune cells in the tumor microenvironment in non-small cell lung cancer, triple negative breast cancer and childhood neuroblastoma in order to identify recurring interactions that could be targeted therapeutically.
Full-length publications
- A. Rybak-Wolf, E. Wyler, T.M. Pentimalli, ..., and N. Rajewsky (2023). Modelling viral encephalitis caused by herpes simplex virus 1 infection in cerebral organoids. Nat Microbiol. https://doi.org/10.1038/s41564-023-01405-y
- T.M. Pentimalli, S. Schallenberg, D. León-Periñán, ..., F. Klauschen, and N. Rajewsky (2023). High-resolution molecular atlas of a lung tumor in 3D. bioRxiv. https://doi.org/10.1101/2023.05.10.539644
Conference presentations
- T.M. Pentimalli, S. Schallenberg, D. León-Periñán, ..., N. Karaiskos, F. Klauschen, and N. Rajewsky. High-resolution molecular atlas of a lung tumor in 3D. (Oral presentation), EMBO Workshop “Cancer cell signalling”, Dubrovnik, Croatia, 16-20 September, 2022.
- T.M. Pentimalli, S. Schallenberg, D. León-Periñán, ..., N. Karaiskos, F. Klauschen, and N. Rajewsky. High-resolution molecular atlas of a lung tumor in 3D. (Oral presentation), Tissue Damage and Healing in Cancer, Berlin, Germany, 23-24 September, 2022.
- T.M. Pentimalli, S. Schallenberg, D. León-Periñán, ..., N. Karaiskos, F. Klauschen, and N. Rajewsky. High-resolution molecular atlas of a lung tumor in 3D. (Poster presentation), AGBT General Meeting 2023, Miami, USA, 6-9 February, 2023.
- T.M. Pentimalli, S. Schallenberg, D. León-Periñán, ..., N. Karaiskos, F. Klauschen, and N. Rajewsky. High-resolution molecular atlas of a lung tumor in 3D. (Oral presentation), 34th Pezcoller symposium “New technologies for studying and treating cancer”, Trento, Italy, 19-20 June, 2023.
- T.M. Pentimalli. 3D molecular reconstruction of a human tumor at single-cell resolution reveals invasion dynamics and predicts mechanism-based, personalized therapeutic targets. (Oral presentation), ISREC-SCCL Symposium 2023: Precision Oncology, Lausanne/Ecublens, 21-24 August, 2023.
- T.M. Pentimalli, S. Schallenberg, D. León-Periñán, ..., N. Karaiskos, F. Klauschen, and N. Rajewsky. High-resolution molecular atlas of a lung tumor in 3D. (Oral presentation), 2nd VIB Conference on 'Tumor Heterogeneity, Plasticity, and Therapy', Leuven, Belgium, 3-5 October, 2023.
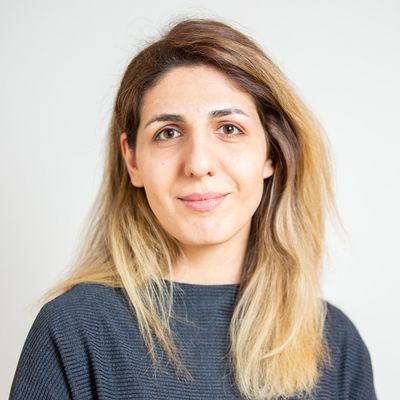
Sepideh Saran
MDC - TU Berlin
Contact
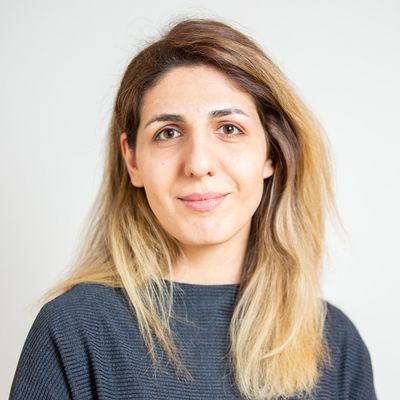
Supervisors:
Advances in experimental methods in Biology and reduced costs of performing high-throughput experiments have provided a vast pool of datasets of various types of measurements. These datasets provide insight into different dimensions of the biological system, including the genome, epigenome, transcriptome, etc. Machine Learning methods can exploit these datasets to study the underlying biological processes, disentangle their causal relationships, and shape new research questions in Biology.
Neural Networks have become the state-of-the-art methods for identifying functional elements in the genome. However, for the ultimate use of these models in many critical downstream tasks, it is essential to be able to explain their decisions and provide a measure for the confidence in the model’s outputs. Experimental noise, incorrect dataset labels, out-of-distribution samples, class imbalance, and the presence of multiple motifs (i.e., multi-label setting) are the major reasons for uncertainty in computational models in Biology. Thus, providing uncertainty measurements, together with model interpretation, enhances the credibility of the proposed machine learning solution and helps clinicians in the subsequent decision-making process.
This project investigates the predictive uncertainty and interpretability of Machine Learning methods in various genomic applications. We focus on tailoring our solution to cope with the limitations of biological datasets, interpretability of the results, as well as model performance and reusability.
Full-length publications
- P. Rautenstrauch, A.H.C. Vlot, S. Saran, and U. Ohler (2021). Intricacies of single-cell multi-omics data integration. Trends in Genetics. https://doi.org/10.1016/j.tig.2021.08.012
Conference presentations
- S. Saran, M. Ghanbari, and U. Ohler. An empirical analysis of uncertainty estimation in genomics applications. (Workshop paper), Bayesian Deep Learning Workshop, NeurIPS 2021, Online, 14 December 2021.
- S. Saran, M. Ghanbari, and U. Ohler. Similarity neural networks for RBP binding site detection (Workshop poster), Learning Meaningful Representations of Life workshop, NeurIPS 2021, Online, 14 December 2021.
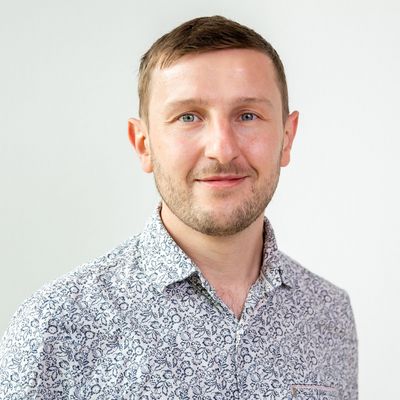
Mario Sänger
HU Berlin
Contact
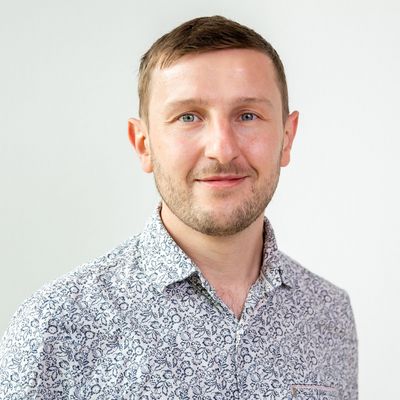
Supervisors:
Researchers are currently producing so many publications that it is impossible to keep up with the boom of discoveries even within a single field. Biomedical information extraction (IE) encompasses methods that aim to automatically collect biomedical knowledge from the scientific literature. These techniques are considered crucial for efficient access to published results at a scale that can cope with scientific progress. IE plays is essential in database curation, the construction of comprehensive models of pathways and cells, and fields such as Personalised Medicine. A key task for IE is the extraction of relationships between entities, such as drugs or proteins that interact with each other in a pathway or cell. While considerable progress in IE has been made over the two decades, there are deficits. Almost all the techniques have focused on extracting relationships from single sentences or single articles.
All sentence- and article-based methods suffer from a number of severe disadvantages in terms of design. First, a single record rarely provides enough evidence to establish the biological validity of a relationship, as the experimental evidence might be weak, or limited to a very specific context. Statements in texts may be more speculative than confirmative, and different articles often contradict each other. Experts therefore usually (a) try to acquire a comprehensive picture of the published state-of-the-art for any given question, and (b) need to include information from other sources in making informed decisions about relationships. There is no consensus on the best way to achieve this automatically. A solution will require finding suitable ways to encode the knowledge contained in large collections of texts and design efficient approaches to integrate different kinds of information (e.g. textual, numerical, categorical and molecular data) that originates from various sources.
This PhD project will contribute to this question while examining, harnessing and combining multiple information sources, such as the entire corpus of literature available through PubMed and additional knowledge base information, in hopes of improving the extraction of information on biomedical relationships. Our approach is fundamentally different than traditional approaches. We classify relations on a global, corpus-based level instead of the sentence- or article-based approaches currently in use. In particular, we want to explore representation learning techniques: instead of explicitly, manually modelling the connections between biomedical concepts, we will apply methods capable of learning adequate representations for these concepts by exploring correlations in large collections of (textual) data.
Full-length publications
- M. Sänger, and U. Leser (2020). Large-scale entity representation learning for biomedical relationship extraction. Bioinformatics, btaa674. https://doi.org/10.1093/bioinformatics/btaa674
- M. Kittner, M. Lamping, D. Rieke, J. Götze, B. Bajwa, I. Jelas, G. Rüter, H. Hautow, M. Sänger, ..., and U. Leser (2021). Annotation and initial evaluation of a large annotated German oncological corpus. JAMIA Open, 4(2), ooab025. https://doi.org/10.1093/jamiaopen/ooab025
- L. Weber, M. Sänger, J. Münchmeyer, M. Habibi, U. Leser, and A. Akbik (2021). HunFlair: An easy-to-use tool for state-of-the-art biomedical named entity recognition. Bioinformatics, btab042. https://doi.org/10.1093/bioinformatics/btab042
- L. Weber, M. Sänger, S. Garda, F. Barth, C. Alt, and U. Leser (2021). Humboldt @ DrugProt: Chemical-protein relation extraction with pretrained transformers and entity descriptions. In Proceedings of the 7th BioCreative Challenge Evaluation Workshop.
- L. Weber, M. Sänger, S. Garda, F. Barth, C. Alt, and U. Leser (2022). Chemical-Protein Relation Extraction with Ensembles of Carefully Tuned Pretrained Language Models. Database. https://doi.org/10.1093/database/baac098
-
J. Fries, L. Weber, N. Seelam, G. Altay, D. Datta, S. Garda, .. , M.Sänger, … , B. Beilharz (2022). Bigbio: a framework for data-centric biomedical natural language processing. Advances in Neural Information Processing Systems, 35, 25792-25806.
-
M. Sänger, N. De Mecquenem, K.E. Lewińska, V. Bountris, F. Lehmann, U. Leser, T. Kosch (2023). Large Language Models to the Rescue: Reducing the Complexity in Scientific Workflow Development Using ChatGPT. arXiv arXiv:2311.01825 [Preprint]
Conference presentations
- J. Seva, M. Sänger and U. Leser. Language-independent ICD-10 Coding using Multi-lingual Embeddings and Recurrent Neural Networks. (Oral presentation), CLEF eHealth 2018.
- M. Sänger, L. Weber, M. Kittner and U Leser. Classifying German Animal Experiment Summaries with Multi-lingual BERT. (Oral presentation), CLEF eHealth 2019.
- M. Saenger, L. Weber and U. Leser. WBI at MEDIQA 2021: Summarizing Consumer Health Questions with Generative Transformers. BioNLP Workshop - MEDIQA, 11 June 2021. https://www.aclweb.org/anthology/2021.bionlp-1.9.pdf
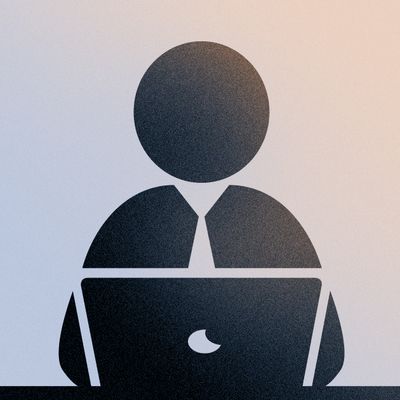
Claudia Winklmayr
MDC
Contacts
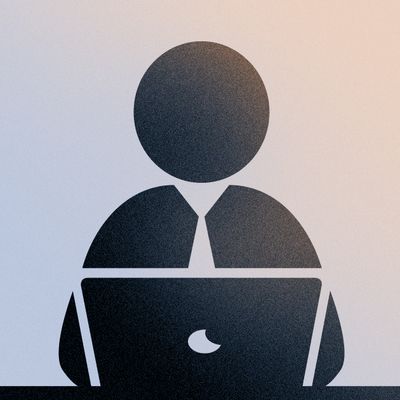
Supervisor:
As a result of the continuous methodological advancement of imaging techniques in the biomedical sciences, practitioners are faced with ever increasing volume of imaging material. The growing demand for high quality image analysis provides ample opportunity for the employment of methods from artificial intelligence (AI), and in particular from computer vision. While these approaches have proven highly successful for a wide range of different tasks, they can sometimes lack robustness or produce overconfident but erroneous results, which has prevented their wide spread application to safety critical domains such as healthcare.
The present PhD project aims at improving upon the robustness and reliability of computer vision methods in the biomedical domain. The main focus lies in the development of concepts and methods for the modeling of uncertainty as well as leveraging approaches from explainable artificial intelligence (XAI) for the use case of instance segmentation. Instance segmentation, a subfield of computer vision, focuses on identifying and delineating individual objects or instances within an image. Despite significant progress over the past years, current methods still require a substantial amount of manual proofreading, which is highly labor intensive, especially in the context of large datasets or 3d imaging data. The results of this project would thus have a direct impact by helping to guide human proofreaders to areas where a predicted segmentation result is uncertain (has high probability of error), suggest potential alternatives and finally provide explanations regarding how a specific segmentation result came about.